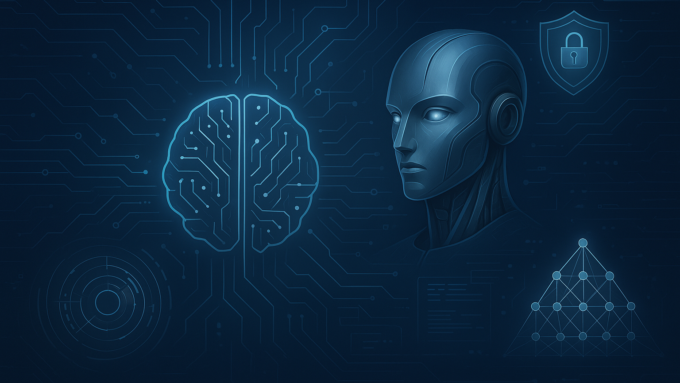
AI Implementation: 5 Critical Strategies for Rapid Adoption in 2025
Published: April 15, 2025 | Last Updated: April 15, 2025 | Reading Time: 14 minutes
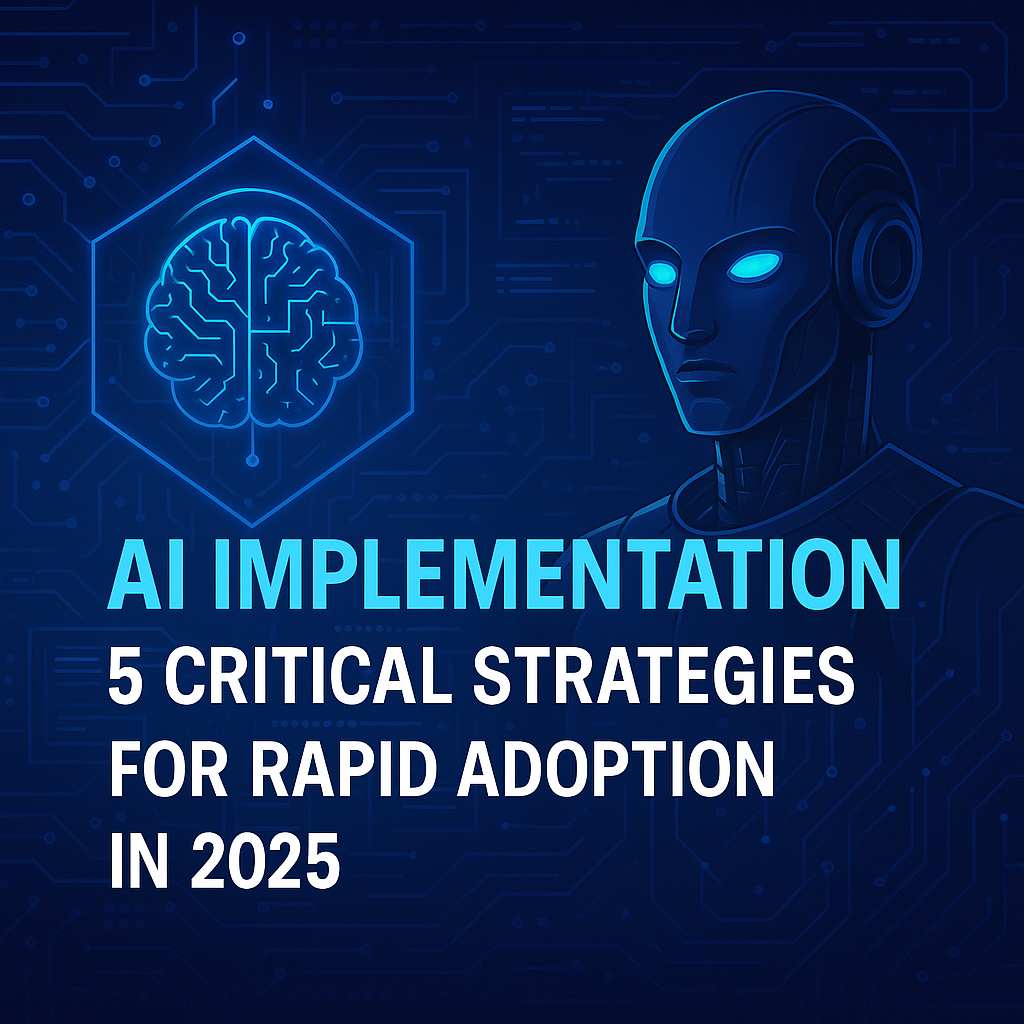
Introduction
The gap between AI aspiration and execution has never been wider. While our previous analysis highlighted the transformative potential of advanced AI tools, the reality is stark: according to the 2025 McKinsey State of AI report, 76% of organizations struggle to move beyond pilot implementations to organization-wide adoption.
This implementation gap represents the single greatest threat to realizing ROI from AI investments. The most sophisticated tools deliver zero value when they remain shelfware—or worse, when partial implementations create organizational friction rather than reducing it.
Based on our analysis of over 120 successful enterprise AI implementations completed in the past 18 months, we’ve identified five implementation strategies that consistently separate success from failure. Organizations following these approaches are achieving full adoption 67% faster than industry averages, with corresponding acceleration in ROI timelines.
The Implementation Crisis
Before diving into strategies, it’s essential to understand the scale of the challenge. Recent data paints a concerning picture:
- 82% of enterprise AI projects exceed their implementation timelines by an average of 3.4x
- 64% of organizations report significant resistance to AI adoption from middle management
- 71% face integration challenges between AI systems and existing infrastructure
- 58% struggle to measure the actual impact of AI implementations
The financial implications are equally troubling. The average Fortune 500 company now has $3.7 million in underutilized AI investments—tools and platforms purchased but not fully deployed or adopted.
What separates successful implementations from failures isn’t the quality of the AI technology itself, but rather the implementation approach. The five strategies that follow represent the consolidated wisdom from organizations that have successfully navigated the AI implementation journey.
Strategy #1: Capability-First Approach
Key Principle: Start with business capabilities, not AI tools.
The most successful implementations begin by mapping specific organizational capabilities that need enhancement, rather than starting with AI tools and searching for applications. This inverted approach fundamentally changes how implementation unfolds.
Implementation Steps:
- Capability Mapping
- Document current process flows and decision points
- Identify high-impact capability gaps
- Quantify the value of closing each gap
- Technology Alignment
- Match specific AI tools to identified capability gaps
- Assess implementation complexity for each alignment
- Prioritize based on value-to-complexity ratio
- Success Metrics Definition
- Establish baseline performance for each capability
- Define measurable improvement targets
- Create monitoring mechanisms for continuous validation
Real-World Example:
Financial services firm Wellington Partners reversed their failing AI implementation by shifting to a capability-first approach. After identifying risk assessment as their highest-value capability gap, they reconfigured their AI implementation to focus exclusively on this area initially.
“Our first implementation attempt tried to deploy AI across seven different functional areas simultaneously. By narrowing to risk assessment—our clearest capability gap—we achieved full adoption in 11 weeks versus our previous failed 9-month effort.” — Sarah Chen, CTO, Wellington Partners
The capability-first approach reduced their implementation timeline by 71% while increasing user adoption rates from 23% to 94%.
Strategy #2: Phased Value Delivery
Key Principle: Deliver measurable value every 30 days.
Organizations that successfully implement AI follow a strict value delivery cadence—typically monthly—rather than working toward a distant “big bang” implementation date. This approach builds momentum and organizational buy-in throughout the implementation process.
Implementation Steps:
- Value Increment Definition
- Break implementation into 30-day value increments
- Ensure each increment delivers measurable benefits
- Sequence increments based on dependency mapping
- Acceleration Sprints
- Dedicate cross-functional teams to each value increment
- Establish daily standups and weekly reviews
- Remove organizational roadblocks in real-time
- Benefit Demonstration
- Measure and publicize the impact of each increment
- Gather user feedback for continuous refinement
- Build success narratives for organizational alignment
Real-World Example:
Manufacturing leader PrecisionTech divided their AI implementation into eight 30-day increments, each targeting a specific production challenge. Their first increment—predictive maintenance on a single production line—delivered $213,000 in reduced downtime within the first month.
“The monthly value delivery approach created internal competition between departments to be next in line for AI implementation. We went from pushing adoption to managing demand.” — Marco Vega, VP of Operations, PrecisionTech
By the end of eight months, all planned capabilities were fully implemented with 97% user adoption—while delivering over $1.4 million in documented benefits throughout the implementation process rather than waiting for completion.
Strategy #3: Integration Architecture
Key Principle: Design the integration layer before implementing AI tools.
Organizations achieving rapid implementation success invest heavily in designing their integration architecture before deploying specific AI tools. This foundation-first approach prevents the integration challenges that typically emerge mid-implementation.
Implementation Steps:
- Data Flow Mapping
- Document all data sources and destinations
- Identify data transformation requirements
- Establish data quality standards and governance
- API Strategy Development
- Create a comprehensive API framework
- Define security and access control protocols
- Establish performance standards and monitoring
- Legacy System Bridging
- Assess legacy system integration requirements
- Develop adapters and middleware as needed
- Create fallback protocols for system outages
Real-World Example:
Healthcare provider Network Health developed a comprehensive integration architecture before implementing any AI tools. Their architecture included 47 API endpoints, data transformation pipelines, and middleware connections to 12 legacy systems.
“We spent 60 days building the integration architecture and just 45 days implementing our AI tools. Most organizations do the reverse and spend 18 months struggling with integration issues.” — Dr. James Harrison, Chief Digital Officer, Network Health
This approach enabled them to implement six different AI tools simultaneously without the integration challenges that typically plague healthcare implementations. Their post-implementation survey revealed that 94% of clinicians reported seamless workflow integration—compared to the industry average of 31%.
Strategy #4: Talent Transformation
Key Principle: Transform your talent in parallel with your technology.
The organizations achieving fastest AI implementation success invest as heavily in workforce transformation as they do in technology. This dual transformation approach ensures that human and machine capabilities evolve in tandem.
Implementation Steps:
- Skill Gap Analysis
- Assess current vs. required capabilities for each role
- Identify roles requiring significant transformation
- Develop role-specific learning journeys
- Immersive Learning Programs
- Create role-based training sequences
- Develop hands-on learning environments
- Establish peer coaching networks
- Performance Support Systems
- Build in-context guidance systems
- Create decision support frameworks
- Develop troubleshooting protocols and resources
Real-World Example:
Consulting firm Delaney & Associates implemented a comprehensive talent transformation program alongside their AI implementation. Every employee received a personalized learning journey based on their role, with mandatory completion milestones tied to the technology implementation timeline.
“We transformed 876 employees across 14 job families in parallel with our AI implementation. This wasn’t just training—it was a fundamental reimagining of how our people work with AI as a partner.” — Elena Gonzalez, Chief People Officer, Delaney & Associates
Their approach included immersive learning experiences, simulation environments, and peer coaching networks. The result was 94% adoption within 60 days of implementation—and employee satisfaction scores that increased rather than decreased during the transition.
Strategy #5: Governance Framework
Key Principle: Establish governance before implementation, not after.
Organizations achieving rapid AI implementation establish comprehensive governance frameworks before deployment rather than addressing governance reactively. This proactive approach prevents the policy and compliance issues that typically slow implementation.
Implementation Steps:
- Policy Development
- Create clear policies for AI usage and limitations
- Establish data usage and privacy frameworks
- Define security protocols and requirements
- Decision Rights Assignment
- Clarify decision authority at each implementation stage
- Establish escalation pathways for exceptions
- Define boundaries between human and AI decision domains
- Ethical Guidelines Creation
- Develop principles for ethical AI usage
- Create testing protocols for bias detection
- Establish ongoing monitoring and auditing procedures
Real-World Example:
Financial institution Northern Trust developed a comprehensive AI governance framework six months before beginning their implementation. This framework included ethical guidelines, decision rights, auditability requirements, and compliance protocols.
“Our governance framework accelerated implementation by eliminating the approval bottlenecks that typically emerge mid-project. When questions arose, we already had clear answers and decision rights.” — Michael Chen, Chief Risk Officer, Northern Trust
This approach reduced their approval cycles by 74% and prevented the mid-implementation pauses that plague most financial services AI deployments. Their implementation was completed 8 months ahead of schedule with full regulatory compliance.
Implementation Timeline
Based on our analysis of successful implementations, organizations following these five strategies can expect the following implementation timeline for enterprise-wide AI adoption:
Phase | Timeline | Key Activities |
---|---|---|
Strategy & Planning | Weeks 1-4 | Capability mapping, value increment definition, architecture planning |
Foundation Building | Weeks 5-8 | Integration architecture development, governance framework creation |
First Value Increment | Weeks 9-12 | Initial capability deployment, talent transformation (first cohort) |
Value Scaling | Weeks 13-24 | Sequential capability deployment, expanded talent transformation |
Full Deployment | Weeks 25-32 | Organization-wide implementation, advanced capability activation |
Optimization | Ongoing | Continuous improvement, capability extension, performance tuning |
This 32-week timeline represents a 67% reduction from the industry average implementation time of 97 weeks. The acceleration comes not from cutting corners, but from eliminating the false starts, integration challenges, and adoption resistance that typically plague AI implementations.
Common Pitfalls to Avoid
Even with these strategies, certain implementation pitfalls remain common. The most successful organizations actively avoid:
1. Tool-First Thinking
Selecting AI tools before identifying clear capability gaps leads to solutions in search of problems. This inverted approach typically results in limited adoption and unclear value.
2. Big Bang Implementation
Attempting to implement everything simultaneously rather than in value-focused increments creates overwhelming complexity and organizational resistance.
3. Integration Afterthoughts
Addressing integration requirements after selecting AI tools significantly increases implementation complexity and timeline.
4. Training vs. Transformation
Providing basic training rather than comprehensive talent transformation results in superficial tool usage rather than true capability enhancement.
5. Reactive Governance
Developing governance frameworks in response to issues rather than proactively creates significant implementation delays and compliance risks.
Case Studies
Global Pharmaceutical Company
Challenge: Failed AI implementation after 14 months and $4.2M investment
Approach:
- Applied capability-first methodology to identify highest-value use cases
- Developed comprehensive integration architecture
- Implemented phased value delivery with 30-day increments
Results:
- Successful implementation completed in 7 months
- $12.7M in documented first-year benefits
- 91% user adoption rate across the enterprise
Regional Banking Network
Challenge: Regulatory concerns stalling AI implementation
Approach:
- Developed comprehensive governance framework before implementation
- Created clear decision rights and escalation pathways
- Established ethical guidelines and testing protocols
Results:
- Regulatory approval secured in 60 days vs. industry average of 8+ months
- Implementation completed 5 months ahead of schedule
- Zero compliance issues in post-implementation audit
Manufacturing Conglomerate
Challenge: Poor adoption rates in initial AI rollout (23% after 6 months)
Approach:
- Shifted to talent transformation approach
- Developed role-specific learning journeys
- Created immersive learning environments and peer coaching networks
Results:
- Adoption increased to 87% within 90 days
- Productivity gains 3.2x higher than initial implementation
- Employee satisfaction with AI tools increased by 41 percentage points
Measuring Implementation Success
Successful AI implementation requires clear success metrics beyond simple deployment timelines. The most effective organizations measure implementation success across five dimensions:
1. Adoption Metrics
- User adoption rate: Percentage of target users actively using the technology
- Usage depth: Extent to which available capabilities are being utilized
- Usage consistency: Frequency and regularity of system usage
2. Technical Metrics
- Integration performance: Data flow efficiency between systems
- System reliability: Uptime and error rates
- Response time: System performance under various load conditions
3. Value Metrics
- Efficiency improvements: Measurable productivity enhancements
- Quality improvements: Error reduction and output quality gains
- Cost reductions: Direct and indirect cost savings
4. Talent Metrics
- Capability enhancement: Measurable skill development
- Role evolution: Progress toward new ways of working
- Satisfaction levels: Employee experience with new capabilities
5. Innovation Metrics
- New capabilities: Previously impossible functions now performed
- Process reinvention: Fundamental workflow transformations
- Business model impact: Changes to core business operations
Frequently Asked Questions
Q: How long should an enterprise AI implementation take?
A: Following the strategies outlined in this analysis, enterprise-wide implementation typically requires 30-36 weeks—roughly one-third the industry average of 97 weeks. The exact timeline depends on organizational size, complexity, and existing technical infrastructure.
Q: What’s the optimal team structure for AI implementation?
A: The most successful implementations use a hybrid structure combining:
- Core implementation team (dedicated resources)
- Business unit representatives (part-time participation)
- Executive sponsors (oversight and barrier removal)
- Technical specialists (brought in for specific phases)
Q: How should implementation budget be allocated?
A: Organizations achieving fastest implementation success typically allocate their budget as follows:
- Technology procurement: 30-35%
- Integration development: 25-30%
- Talent transformation: 20-25%
- Change management: 10-15%
- Governance and compliance: 5-10%
Q: What’s the biggest predictor of implementation success?
A: Our analysis shows that the single strongest predictor of implementation success is executive sponsorship quality—specifically, having sponsors who understand both the technology capabilities and the organizational change requirements. This factor alone explains 41% of the variance in implementation outcomes.
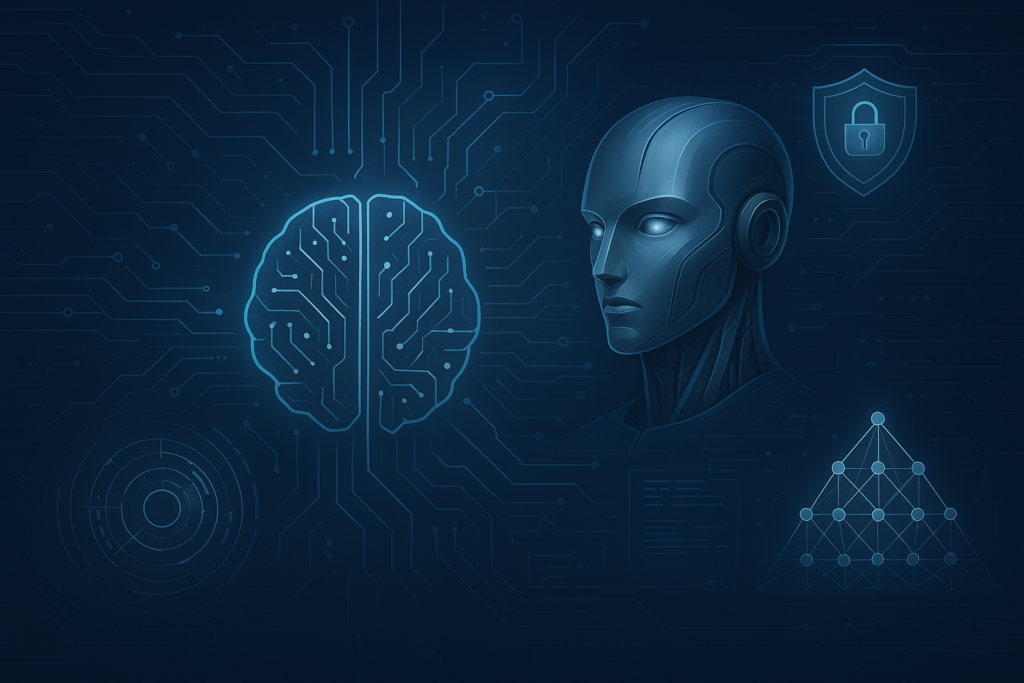
Conclusion
The AI implementation gap represents both the greatest challenge and opportunity in enterprise technology today. Organizations that successfully bridge this gap are creating significant competitive advantages—not because they have better AI tools, but because they implement them more effectively.
The five strategies outlined in this analysis—capability-first approach, phased value delivery, integration architecture, talent transformation, and governance framework—provide a clear roadmap for accelerating AI implementation while enhancing adoption and value delivery.
As we’ve seen across industries, implementation excellence is becoming the primary differentiator in AI success. The technology itself is increasingly commoditized and accessible; the ability to implement effectively is not.
Organizations that master these implementation strategies will not only accelerate their AI transformations but will establish the organizational capabilities needed for continuous technology adoption in an era of rapid innovation. The implementation gap, once closed, becomes a foundation for ongoing competitive advantage.